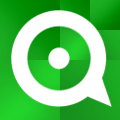
Regression modeling is undergoing a revolution precipitated by the availability of hundreds and even thousands of candidate predictor variables in genomics, but increasingly vast amounts of data are becoming available in all other fields as well. Problems in traditional regression modeling occur when the number of predictors P included in a model approaches or exceeds the sample size N. In this type of situation, involving the presence of ‘high-dimensional data’, traditional regression methods become unreliable and regression coefficients may even be impossible to estimate. Recent advances with high-dimensional data show how such problems can be resolved (see: Cai and Shen (2011)). This important new field continues to evolve at a rapid pace.
Statistical Innovations is pleased to announce our first new software innovation since Latent GOLD in 2000! CORExpress focuses on regression analysis (linear regression, logistic regression, etc.) where large numbers of correlated predictors may be available. On many data sets, it has been shown to outperform penalized regression techniques such as Lasso, and other methods such as Naive Bayes and PLS regression.
Comments